What is A/B testing?
For marketers, data has become increasingly important. Gone are the days where guesswork was sometimes used. More recently, data has been used as a way to guide our decision making and move away from expensive mistakes.
One common and successful method of getting new data is A/B testing. Also called Split testing.
What is A/B testing?
An A/B test is an experiment that compares two variables with each other. You randomly assign either variable to separate audiences over a pre-determined amount of time. As long as the experiment is controlled and not biased, an A/B split test is a great way to find out which version of a product or website is better.
The process of conducting an A/B test is:
- Form the hypothesis
- Test the hypothesis
- Analyse the data
- Conclude a result
- Take action based on the result
- Repeat this process
So how do we do a split test?
An A/B test is a test that can run on many different variations. You can test email subject lines, website text, button locations and much more.
Let’s use the example of button placement.
- Hypothesis: If we move the button above the fold we will increase our clickthrough rate by 10%
- Variable A: Keep the button in the same location
- Variable B: Move the button above the fold
- Sample size: 10,000 website visitors
- Duration: at least 3 month
In the example above, Variable A is the original and variable B is the challenger.
Typically, we recommend running A/B tests for at least 3 months. The more data you have, the better.
Make sure you analyse the data correctly.
It is important to have the correct information when you are running your checks. For example, if you have a very slow month in March, usually, it is very normal that your results will show a bit of a skew. This is important information to keep in mind.
It is important to make sure that your information compares as much comparable information as possible. Not having apples and oranges as a comparison, but apples and apples.
Make sure that your data set is as large as possible. This provides you with as much relevant and accurate information as possible.
From the blog
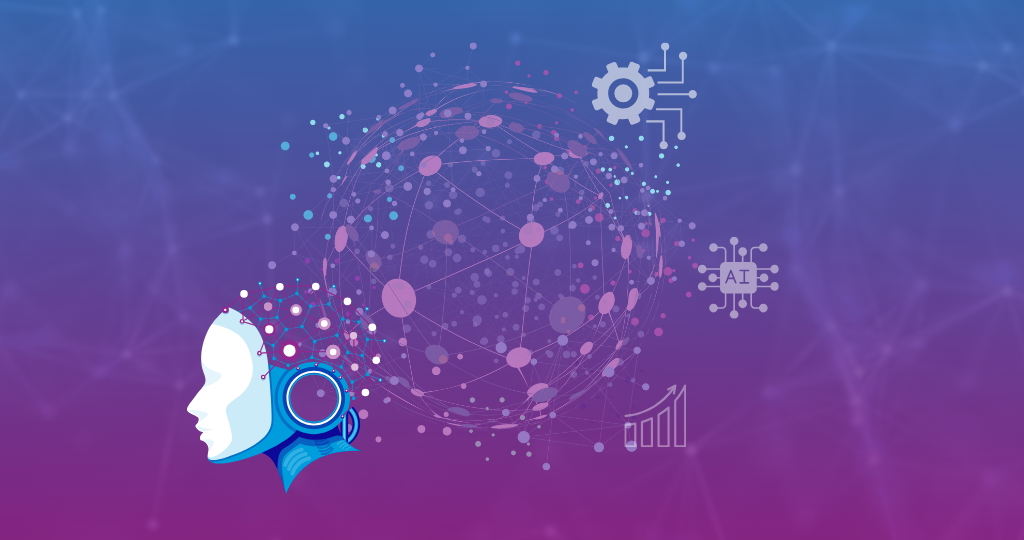
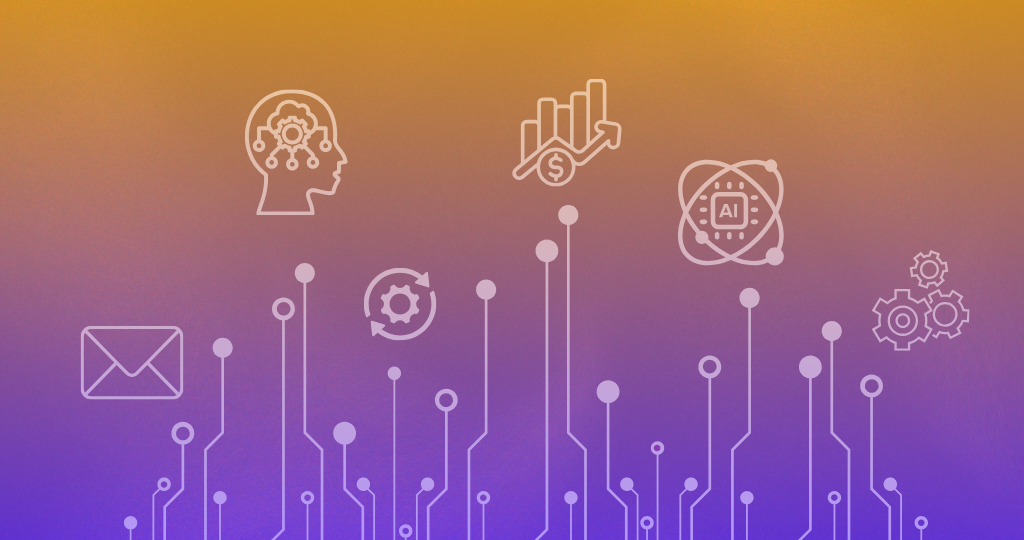